Article
Understanding AI Risk Management Banking
Learn how AI risk management and other AI-powered automation tools are used by banks to improve fraud detection, model credit risks, and strengthen compliance management in the financial industry.
- Loading table of contents...
Examples of risk in the banking industry
As people around the world live increasingly digital lives, businesses are under new pressure to adapt systems and processes to simultaneously improve speed and lower costs. The financial services industry, and banking sector, in particular, are at the very forefront of this transformation, leveraging emerging technologies such as artificial intelligence (AI) and machine learning (ML) to reduce fraud, unlock new efficiencies, and better serve the customer.
In this post, we take a detailed look at how AI and other forms of intelligent automation can be used to reshape the banking industry, particularly as it relates to risk management.

Examples of risk in the banking industry
The banking industry is continuously exposed to a variety of risks, the most common of which include:
AI risk management technology for the banking industry

The financial services sector typically works with vast amounts of data – the likes of which has exploded in recent years and is expected to balloon even further in those to come. It is estimated that in 2020, every human created at least 1.7 MB of data per second on average; by 2025, society as a whole is expected to produce 463 exabytes of data every day worldwide.
Within these large, complex data sets are insights that banks can use to develop more accurate risk models, improve decision-making and identify suspicious activity or transactions in real time. However, humans alone do not have the capabilities to analyze this quantity of data accurately. As a result, banks are turning to technology to effectively process vast amounts of data and identify key insights that can improve decision-making, reduce risk and enhance the customer experience.
1. Artificial intelligence (AI)
AI leverages algorithms to analyze large, complex data sets at scale and identify patterns and predict outcomes. This helps banks mitigate risk more effectively by detecting suspicious or anomalous activity that indicates the presence of fraud.
2. Machine learning (ML)
ML is a form of intelligent automation that leverages models to analyze existing data and accurately forecast outcomes based on patterns. ML algorithms can be used to review vast quantities of data, identify correlations, calculate risk and inform decision-making.
3. Deep learning
Deep learning uses neural networks to learn from big data sets. Unlike ML, which uses predefined models to identify patterns, the neural networks in deep learning tools imitate the behavior of the human brain, using reasoning to identify patterns or anomalies. In the banking industry, deep learning is used to more accurately calculate credit risk for loan or credit card applicants, as well as identify trends or predict events that can impact the creditworthiness of an entire group.
4. Natural language processing (NLP)
NLP is a type of AI that enables computers to “read” copy and understand its meaning. In the banking industry, organizations can leverage NLP to extract relevant information from a structured or unstructured data set. This can greatly improve the speed at which banks can process applications, open new accounts, resolve customer service requests, onboard new employees or complete any other task that requires the organization to gather and synthesize text-based information.
5. Intelligent document processing (IDP)
IDP is a form of intelligent automation that leverages advanced technology to extract semi-structured or unstructured data from files and convert it into structured, usable data. Intelligent document processing enables enterprises to eliminate manual data processing tasks, which greatly improves processing times, reduces costs, and eliminates errors.
6. Big data analytics
Banks use specific technological tools to analyze large data sets with the goal of identifying patterns, correlations, and market trends. Although these tools do not necessarily use AI capabilities, the technology allows banks and their IT teams to better understand and mitigate risk.
Key use cases of AI in risk management for banks
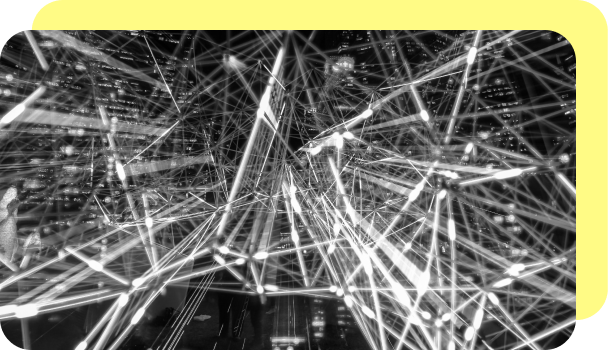
There are several ways that AI and related technologies have allowed banks to identify and reduce risk in a more effective and timely manner. These include:
1. Fraud detection
Loan application fraud detection: ML algorithms can help banks identify inconsistencies and discrepancies in loan applications. In our current digital landscape, fraudsters to carry out identity theft to submit fraudulent loan or credit applications. Without the help of AI- or ML-enabled models, banks would struggle to find the balance between processing applications quickly and ensuring the application and its documentation are accurate.
Real-time transaction fraud detection: Manual fraud detection systems, which rely largely on human analysts, are no longer a practical or effective solution for keeping up with the explosion of digital transactions occurring in the modern landscape. AI-enabled risk management tools and techniques offer organizations real-time data analysis, enabling them to block potentially fraudulent transactions or require verification before proceeding.
In the case of both loan fraud detection and transaction fraud detection, the AI or ML model will become more intelligent over time. Meaning, that as models take in more and more data, their analysis capabilities become more precise, which helps them become more accurate over time and reduces the number of false positives within the system.
2. Regulatory compliance management
Complying with national and international regulations is an extremely important and complex activity within the financial sector. Large data sets are required to be reviewed, numerous variables need to be analyzed, and accurate documentation submitted to the appropriate authorities. Machine learning automates these activities and ensures they are completed efficiently and accurately. This unlocks significant cost savings for banks in the form of resource optimization and avoidance of fines or penalties. ML technology can also decrease the number of false alerts in the compliance system, ensuring issues are processed manually by a human only when necessary.
3. Credit risk modeling
Traditional banks use AI, ML, and deep learning to assess each person’s individual credit risk. These models and neural networks leverage a wide variety of inputs, including personal information, historical behavior data, and the person’s existing assets, as well as external factors and trends that may impact their ability to repay a loan, to produce a personalized risk score.
4. Insider threat prevention
AI and related tools and technologies are also used to monitor the activity of traders and other financial professionals to identify early signs of fraud, insider trading, data theft, or other forms of financial crime and wrongdoing. AI-enabled tools are able to log, monitor, and analyze phone logs, email traffic, time cards, and vacation schedules, to identify and alert the organization to potential risks and misconduct.
Key Benefits to AI in banking risk management
Using AI in financial risk management unlocks an array of key benefits in the banking industry, including:
- Lower operational costs and efficiency gains through process automation and resource optimization
- Improved compliance through automated monitoring and reporting
- More timely, accurate and personalized risk assessments and credit risk scores through advanced data analysis
- Reduced opportunity for human error through process automation
- An enhanced customer experience through data-enabled personalization
Are AI-powered risk management solutions right for me?
Financial risk is best mitigated with the right tools and technologies. At Inscribe, we help banks manage risk by enabling teams to detect fraud, automate processes, and better understand customer creditworthiness.
What we offer:
- AI-powered fraud detection to enable high-trust online use cases for the financial services industry. Over the past year, we have onboarded some of the world's fastest-growing fintechs and numerous Fortune 500 companies, helping them to reduce fraud and give quicker decisions to their customers.
- Intelligent document automation to improve processing time and accuracy of customer applications by extracting key fields from documents including bank statements, transactions, and payslips. We work with clients to help reduce manual processes and integrate our easy-to-use API to automate document checks, streamline account opening or underwriting processes, and enhance compliance.
- Actionable insights and analysis to better understand your customers’ creditworthiness and make data-driven decisions with AI-powered insights.
To learn more about how Inscribe can help your bank, credit union, insurer, or other financial services organizations, please schedule a personalized demo today.