Article
Credit Card Fraud Detection: A Guide
Credit card fraud detection has become more important than ever. Check out this in-depth article to learn what is credit fraud and everything you need to know about it.
- Loading table of contents...
Losses related to credit card fraud will grow to $43 billion within five years and climb to $408.5 billion globally within the next decade, according to a recent Nilson Report — meaning that credit card fraud detection has become more important than ever.
The sting of these rising costs will be felt by all parties within the payment lifecycle: from banks and credit card companies who foot the bill of such fraud, to the consumers who pay higher fees or receive lower credit scores, to merchants and small businesses who are slapped with chargeback fees.
With digital crime and online fraud of all kinds on the rise, it's more important than ever for organizations to take firm and clear steps to prevent payment card fraud through advanced technology and strong security measures.
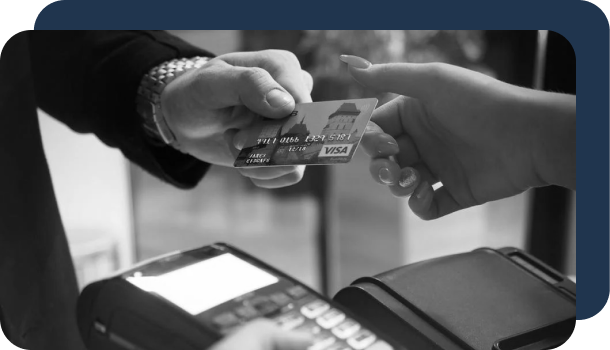
What Is credit card fraud?
Credit card fraud is the act of using another person's credit card to make purchases or request cash advances without the cardholder's knowledge or consent. These criminals may obtain the card itself through physical theft, though increasingly fraudsters are leveraging digital means to steal the credit card number and accompanying personal information to make illicit transactions.
There is some overlap between identity theft and credit card theft. In fact, credit card theft is one of the most common forms of identity theft. In such cases, a fraudster uses an individual's personal information, which is often stolen as part of a cyberattack or data breach, to open a new account that the victim does not know about. This activity is considered both identity fraud and credit card fraud.
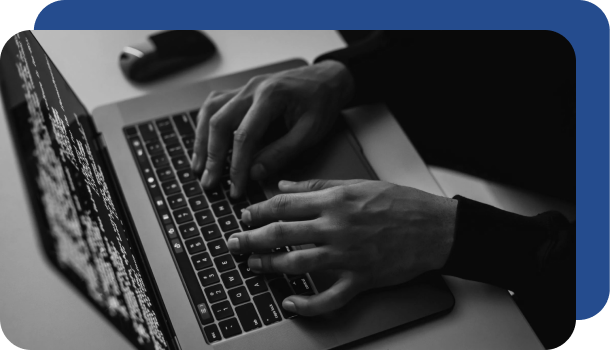
Types of credit card fraud
Credit card fraud falls into two basic categories:
- Card present fraud
- Card-not-present fraud
Card present fraud
Card present fraud is when the criminal uses a physical card, which is either stolen or duplicated, to make fraudulent purchases. Card present fraud can be the result of the theft of a card through robbery, pickpocketing, or mail theft.
Criminals may also leverage card skimmers installed at frequently used payment points to collect and store the card details when swiped; this data can then be used to produce a duplicate payment card, or clone.
Card-not-present fraud
Card-not-present fraud is when the criminal uses the details associated with the card, such as the card number, accountholder name, and CVV code, without having the card in their possession.
In some cases, card-not-present crime is accompanied by account takeover techniques. This is when fraudsters contact a credit card issuer and purport to be a legitimate card holder to change information associated with the account, such as a phone number or address. This will allow them to verify purchases and authenticate activity, thereby evading many fraud detection tools.
Advances in card security
Since 2015, credit card fraud has shifted dramatically toward card-not-present crime. This is largely because of EMV (Europay, MasterCard and Visa) chip technology introduced by major credit card companies around the world.
Sometimes referred to as chip and PIN, EMV equips credit and debit cards with a microchip that can be inserted into a card reader or scanned via contactless payment method to process the transaction. This method is far more secure in that criminals can no longer use card skimmers to capture data from the magnetic strip of the card and produce a clone.

At the same time, massive data breaches at various credit institutions, retailers and other businesses have created a black market for compromised credit card data and sensitive information. Cybercriminals that have stolen this information often post it on the dark web, making it available for purchase by other individuals. The availability of compromised card information, coupled with a rise in online shopping, has dramatically increased card-not-present credit card fraud.
At present, there is no technological solution similar to an EMV microchip that can significantly assist in card-not-present fraud prevetion for credit cards. Instead, the burden is on both credit card issuers and merchants to employ stronger digital security measures to identify and block fraudulent transactions in real-time.
What is credit card fraud detection?
Credit card fraud detection is the collective term for the policies, tools, methodologies, and practices that credit card companies and financial institutions take to combat identity fraud and stop fraudulent transactions.
In recent years, as the amount of data has exploded and the number of payment card transactions has skyrocketed, credit fraud detection has become largely digitized and automated.. Most modern solutions leverage artificial intelligence (AI) and machine learning (ML) to manage data analysis, predictive modeling, decision-making, fraud alerts and remediation activity that occur when individual instances of credit card fraud are detected.

Anomaly detection
Anomaly detection is the process of analyzing massive amounts of data points from both internal and external sources to produce a framework of "normal" activity for each individual user and establish regular patterns in their activity.
Data used to create the user profile includes:
- Purchase history and other historical data
- Location
- Device ID
- IP address
- Payment amount
- Transaction information
When a transaction falls outside the scope of normal activity, the anomaly detection tool will then alert the card issuer and, in some cases, the user. Depending on the transaction details and risk score assigned to the action, these fraud detection systems may flag the purchase for review or put a hold on the transaction until the user verifies their activity.
What can be an anomaly?
- A sudden increase in spending
- Purchase of a large ticket item
- A series of rapid transactions
- Multiple transactions with the same merchant
- Transactions that originate in an unusual location or foreign country
- Transactions that occur at unusual times
If the anomaly detection tool leverages ML, the models can also be self-learning, meaning that they will constantly gather and analyze new data to update the existing model and provide a more precise scope of acceptable activity for the user.
It is important to note that the most advanced algorithms are based on the individual user's behaviors and transaction history. Therefore, the model could make exceptions and allow transactions that are usually considered high-risk for the wider user base.
For example, for a business executive who travels internationally frequently, a rule may allow her to make routine purchases in select countries where she has a history of similar activity. On the other hand, a cardholder who rarely leaves their state likely will not be able to make any international purchases without first alerting their bank to upcoming travel plans.
Predictive modeling
In addition to finding anomalies within a specific user account, ML models and predictive analytics can also be used to track and identify fraud patterns or point to an ongoing, nuanced fraud scheme. Predictive modeling is an important capability since cybercriminals are constantly updating their techniques to evade detection by existing tools and methods.
Outlier models
Finally, some anomaly detection tools are also equipped with outlier models. As the name implies, an outlier model identifies suspicious activity in the cases when not enough data is available to predict patterns.
For example, if a user has only used their debit card to make small, regular purchases within their local area and the card is suddenly used to purchase a large ticket item, that could indicate fraud. In this case, the tool would flag the transaction for further review, or request the credit card holder confirm their purchase before processing the transaction.
Combating fraudulent activities with Inscribe
At Inscribe, we're on a mission to create a more fair and efficient financial services ecosystem. That's we've built best-in-class document fraud detection software, and then laid our document intelligence and processing automation features on top of that.
Our suite of 20+ fraud detectors examine the metadata, pixel-level information, and more in documents to ensure the integrity of the information presented. Additionally, we compare documents to templates of known fraudulent and known legitimate documents. Detecting fraud in documents is Inscribe's competitive advantage in the market for risk management.
Over the past year we have onboarded some of the world's fastest growing fintechs and numerous Fortune 500 companies, helping them to reduce fraud and give quicker decisions to their customers. In April 2021, we surpassed $40M of fraud detected per month for our customers. And we've helped our customers upload and analyze thousands of documents for fraud accurately in just seconds.
Want to learn more about how Inscribe can help your organization combat fraud? Check out our recent article about new features we released to help our customers fight fraud, or contact our sales team to schedule a personalized demo.